Originally published by Southern Voice January 21, 2019.
By Emmanuel Letouzé and Micol Stock
The development effectiveness landscape has been significantly transformed since the two major milestones of the Paris Declaration on Aid Effectiveness in 2005 and the 2008 Accra Agenda for Action. On the political economy side, the transition from the Millenium Development Goals (MDGs) to the Sustainable Development Goals (SDGs), and the recent rise of political polarization and populism, disclose and fuel new concerns. On the techno-scientific side, major
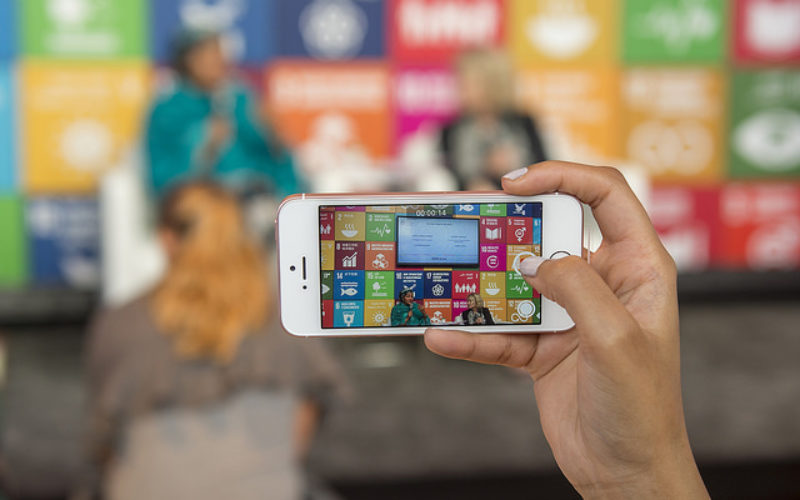
change drivers have emerged. For example the spread of Randomized Control Trials (RCTs), and, of course, the growing use of digital devices, services, and data.
Big Data in development
Big Data creates opportunities for quicker and more targeted information and decision making. But it can also lead to divisions, distrust and overconfidence in the power of technological fixes. In this context, the question of whether and how Big Data could help donors, policymakers, and development professionals to get a better sense of the effectiveness of development—particularly of Official Development Assistance (ODA), South-South Cooperation, and Blended Finance— has become more relevant.
The improvement of predictive machine learning models, for example, has given a new impetus to an old debate about the balance between formative and summative evaluations. There are calls for a greater focus on “improving” over “proving”. In parallel to this, evaluation experts increasingly recognize the growing complexity of both the interventions to be evaluated and the contexts within which they are deployed.
One important insight is that Big Data has contrasting effects on the “evaluability challenge”. This means: the extent to and ways in which causality can be credibly assigned between an aid-funded intervention and the observed outcomes. It can analyse, for example, the impact of a new transportation system on economic opportunities and citizen security. On the one hand, new data and tools can lead to new insights on human processes (such as fine-grained mobility or poverty estimates), including from quasi-natural experiments. On the other hand, the many feedback loops they create, may further complicate causal inference. With so much data to crunch, this could tempt to bypass careful scientific design.
In response, a consensus has emerged for the use of mixed methods. It includes a qualitative and a quantitative analysis. Being more adaptive, they are increasingly ‘embedded’ in daily processes and allow more dynamism, richer sets of indicators and substantiated feedback that may identify unintended consequences. Guidelines have been developed to integrate Big Data into Monitoring and Evaluation (M&E) of development programmes.
The new normative landscape is testing standard practices and opening up new avenues. For example, some experts question that the standard DAC criteria for Evaluating Development Assistance can adequately capture the new values and objectives embedded in the SDGs and post-2015 agenda (to which the Principles for Digital Development could be added). Social inclusiveness and environmental sustainability ought to challenge the praxis, metrics and timeframes commonly used to determine effectiveness. An area where new goals and tools meet is the measurement of Tier3 SDG indicators, for which no methodology has yet been formalized. For example Data-Pop Alliance and UNDP have recently launched a pilot programme to estimate the ‘Percentage of population satisfied with their last experience in public services’ in Botswana. They do this by extracting and analyzing large sets of information from social media.
Artificial Intelligence & aid effectiveness
Artificial Intelligence (AI) is also poised to affect aid effectiveness. AI applications analyze and categorize large amounts of text and pictures. They produce and connect relevant datasets based on a simple differentiation between images and objects, and the identification of people and groups. Machine learning models are also used to improve the effectiveness and fairness of social programs. AI can predict false positives (people who benefit but shouldn’t) and false negatives (people who don’t benefit but should). These approaches require having access to appropriate data, often collected by private organizations, in a reliable, predictable, and ethical manner. This will take efforts that development experts are familiar with, such as building trust, partnerships, data systems and baselines. But it will be with new stakeholders and new incentives.
The Open Algorithms (OPAL) project may facilitate the transition to greater reliance on these ‘private’ data. It is currently deployed by a consortium of partners, in collaboration with two leading telecom operators in Colombia and Senegal. OPAL aims at extracting key indicators (such as population density, poverty, or diversity) through a secured open source platform. It also relies on open algorithms running on the companies’ servers, behind their firewalls. OPAL comes with governance standards that ensure the security, auditability, inclusivity, and relevance of the algorithms for different scenarios.
AI systems can also provide a useful ‘aspirational analogy’ to make future human actions more effective. What makes current AI so impressively good at its job is the credit assignment function. The ability of the algorithms to identify and reinforce the Artificial Neural Networks that most contribute to coming up with the “right’ result through many iterations and data-fueled feedback loops. These allow for machine learning. In a future ‘Human AI ecosystem’, governments, corporations or the aid sector, could apply AI tools to identify and reinforce what contributes to ‘good policy results’, including outcomes of aid programs. They could also better understand whether these effects are desirable in the long run through feedbacks.
Building such Human AI ecosystems will require a few key social and technological features: a healthy data culture, with widespread data literacy. This means for stakeholders that they have to demand evidence-based policies. There should be incentives to request that the effectiveness of publicly financed programmes be assessed using the best available data and methodologies.
“Improve” rather than “prove”
In complex development contexts, it is especially hard to assign causality and draw conclusions on the effectiveness of financial flows. Big Data can complement conventional evaluation methodologies. It provides cheap, quick, complexity-sensitive, longitudinal, and easily analyzable information. As AI keeps evolving, its relevance to M&E will likely grow as well.
In an ever-more digital world, ‘Human AI’ systems fueled by data-backed evidence will point to which actions are likely to improve (rather than prove) outcomes. These will be key to effective development programming in the future.
Read more
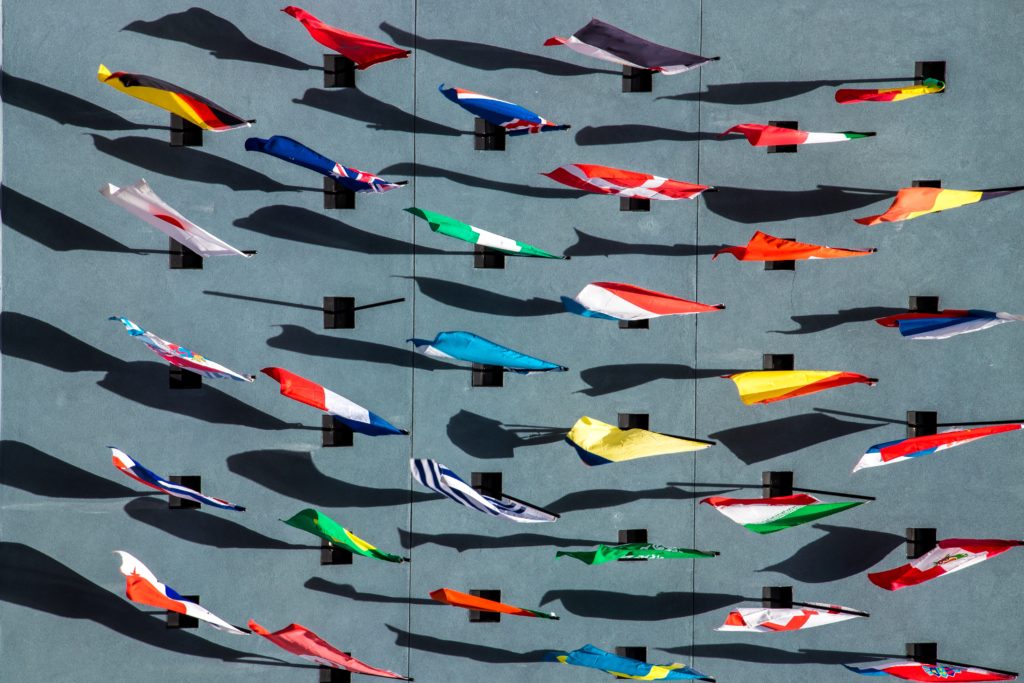
Measurement and Development
Data-Pop Alliance has ongoing research and programs around measurement and Sustainable Development. Our work in this field aims to contribute to the international community’s debate on the role of innovative methodologies, including new approaches based on artificial intelligence and Big Data, in ensuring global accountability towards the UN Agenda 2030 for Sustainable Development. Our research is presented in papers, focused discussions and workshops.