LINKS WE LIKE #26
The rapid expansion of Artificial Intelligence (AI) models has been accompanied by growing efforts to make these technologies accessible and available to non-experts through what is referred to as open-source licenses. As a result of this “democratization of AI” more models and training data are becoming public, a process that has given rise to its new possibilities and challenges.
The OpenAI’s GPT-3 language model, freely accessible to everyone, has become a beacon for the potential use of AI. Despite this, it is still undergoing improvements and “responsible AI” considerations. Tech companies are also joining this movement by making their AI platforms available for researchers, NGOs, and individuals. AT&T, for instance, is allowing its employees to access their Machine Learning (ML) Platform, which facilitates the use of AI components-such as natural language processors-to build their own AI applications. Furthermore, companies like DataRobot Inc. and Petuum have also developed ML platforms that enable users to build predictive models and applications using AI techniques.
Alongside the creation and opportunities of greater accessibility to these models, the debate of under which licenses should AI models be released remains. As academics have noted, there is a big concern regarding issues such as privacy, intellectual privacy rights, and potential harms to marginalized communities that have made AI accessibility a highly complex and contentious issue. Therefore, when releasing an open-source algorithm for an AI model, it is important to understand the guarantees and limitations that come with the chosen license. There are a variety of licenses ranging from strong copy-left licenses (more restrictive in its terms) like GPLv3, AGPL, to more permissive ones like the Apache license 2.0 or the MIT license. Yet, due to the open nature of the licenses, there is limited control over what a developer decides that others can do with a shared code and model.
While specialized licenses such as Responsible AI Licenses have been created to hamper potential harmful applications of AI and Machine learning software, there is still a long way to go for ensuring ethical use of AI and developing models that are accessible to everyone. This month’s Links We Like covers this issue and the debates around it by exploring resources about different AI licenses and ways in which training data and models are being designed to become more approachable.
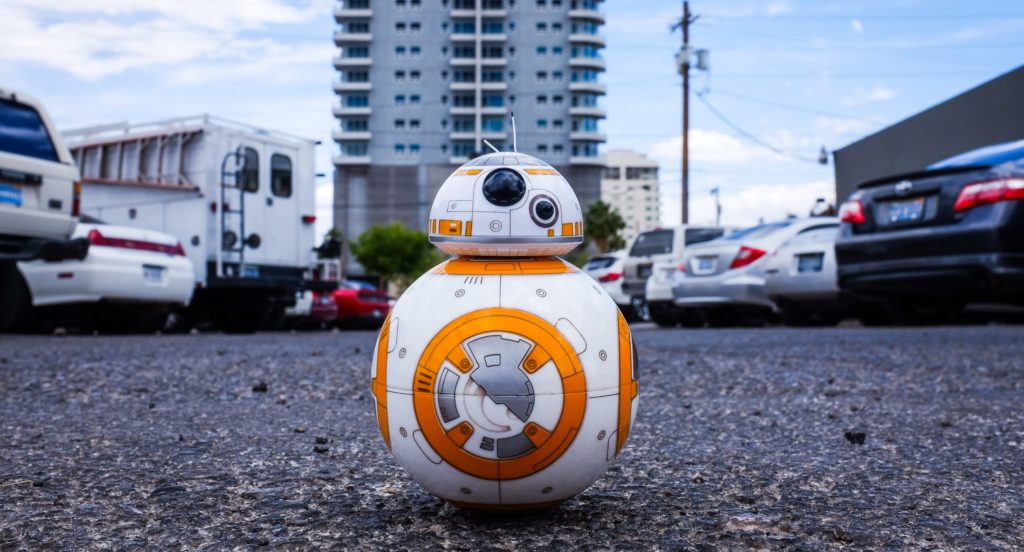
In their article, director of policy Brigitte Vézina and senior counsel, Sarah Pearson from Creative Commons, raise the question of whether CC-Licensed content can be used to train AI. They begin by specifying that this debate is still open and the answer is uncertain. However, the author’s stance as part of Creative Commons is that the use of work to train AI should be considered non-infringing by default, as long as access to the copyright works are lawful. Nonetheless, there is still much caution regarding this issue. Companies like IBM have tried training their facial recognition AI programs by feeding their algorithms with CC-licensed photos in collections that were made public but did not have permission from the people photographed. This incident increased the tension between the value of open data and the ethical-moral use of open licensed content. The conclusion, for now, is that we need to continue having conversations regarding this topic and to be involved in initiatives such as the CC Copyright Platform to plan and coordinate the ethical standards necessary for copyright law and policy-related activities when using AI models.
Through his blogpost Vadym Kublik, Data Protection Manager at AIESEC in Finland, explains how data used to train Machine Learning (ML) algorithms can be restricted by Copyright Laws. He asserts that only public domain content is harmless to use in ML projects. However, the majority of creative works, such as images, literary works, music, and others are protected by intellectual property law (IP Law) such rules must be considered before using data to train algorithms. The article further highlights that while creative works are usually shared through licenses such as Creative Commons licenses –which are considered to be fairly open and are viewed as low-risk in its use for AI–, data and privacy regulations vary from jurisdiction to jurisdiction. These differences between legal frameworks represent an immense challenge for ML researchers given that each piece of data may have to be managed differently.
It can’t be denied that significant advances have been and are being made in AI, and yet, accessing and taking advantage of Machine Learning (ML) systems can raise fundamental AI, ethics, and copyrights concerns. In her blog post, Karen Robinson tries to unpack ML copyrights nexus and whether the use of protected material in the process of training an AI model constitutes infringement. According to Robinson, the answer lies in the fair use doctrine. The latter means that if copyrighted material reduces the market value of the work to its original creator, it is unlikely to be considered fair use. Although using copyrighted material in training algorithms does not diminish the economic value of the work in any measurable way, the answer to this question is not straightforward given the lack of legal frameworks regulating intellectual property and ML in the United States. Few countries (e.g. Japan) updated their legal frameworks to include exemptions of the use of copyrighted works for machine learning. IBM’s CEO raised the question of the need for a ‘precision regulation’ to overcome this challenge.
Copyright laws in AI can be problematic to the point of further contributing to discrimination. This situation arises from the fact that these laws limit access to necessary training data (often for legitimate reasons) “forcing” AI creators towards using what is referred to as Biased, Low- Friction Data (BLFD) to train models. Amanda Levendowski investigates this issue on a deeper level in her paper where she discusses how copyright laws and licensing could become channels for promoting biased AI systems. She explains that most programmers avoid infringing copyright laws and are resolute to use BLFD to build their models. The matter remains legally in a grey area, and courts are unable to pinpoint datasets used for training AI systems as necessarily infringed copies of original human work. Consequently, these rules are causing two types of friction: competition and access. Competitively, these laws make it harder to implement bias mitigation processes or create less biased programs. In terms of access, the law privileges some individuals’ work over others’, which again, will encourage AI creators to use more easily available, and usually highly biased datasets. Despite these limits the author concludes by showing that not all copying is legally considered infringement. In fact, she also proposes the fair use doctrine (see article above) his doctrine provides a flexible way through which the interests of both the copywriters and AI creators remain protected.
The OECD created an interactive AI Policy Observatory where they gather different information regarding AI policies, news, and initiatives from different countries around the world. The observatory contains data and multi-disciplinary analysis on artificial intelligence that is nurtured by the information provided by a diverse global community of partners in 60 countries. Due to the importance national legislation has in the framework for creating and developing AI models, the observatory contains an interactive database of AI policies and initiatives from countries, territories, and other stakeholders to facilitate cooperation at an international level. The platform also contains an AI-powered tool that displays real-time information on COVID-19 developments per country. Check it out by clicking the link in the title.
Further Afield
AI, Machine learning & Copyrights
- IBM CEO Ginni Rometty calls for ‘precision regulation’ on AI
- Calls for AI Regulation Gain Steam
- AI, Ethics and Copyright – Hugh Stephens Blog
- Artificial Intelligence and its challenges for Intellectual Property
- Is Intellectual Property Law Ready for Artificial Intelligence?
- Copyright Law Should Not Restrict AI Systems From Using Public Data
- WIPO draft issues paper on intellectual property and artificial intelligence Response from the British Copyright Council
Machine learning & accessibility
AI Licenses
- Use and Fair Use: Statement on shared images in facial recognition AI
- Using Creative Commons images to train artificial intelligence
- Creative Commons says copyright can’t protect your photos from ending up in a facial recognition database
- Towards Standardization of Data Licenses: The Montreal Data License
- Open Source Licenses in Machine Learning and Self-driving Car Projects
- Open source licenses: Which, What and Why
- An open source license that require users to do no harm
Training Data and AI Models
- How Training Data in Machine Learning is Used to Develop an AI Model?
- Top 8 open source AI technologies in machine learning
- Microsoft teams up with OpenAI to exclusively license GPT-3 language model
- Facial recognition technology can expose political orientation from naturalistic facial images
- Facial recognition technology can expose political orientation from naturalistic facial images
- Can open-source innovation accelerate AI development?
- Developing artificial intelligence tools for all
AI Strategies