OPAL for Public Data and Good
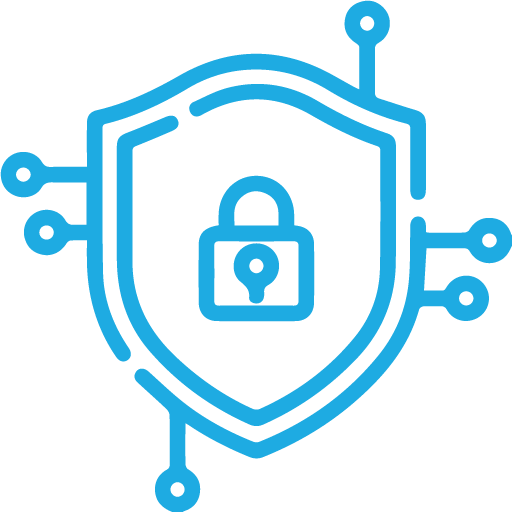
What?
OPAL (for Open Algorithms) is a socio-technological innovation designed to unleash the power of sensitive data in a privacy-preserving, participatory, ethical, sustainable and scalable manner.
How?
By allowing for the use of data such as mobile phone records, financial transactions, census and survey microdata, to be collected by private and public entities (e.g. telecommunications operators, financial companies, National Statistical Offices).
Why?
To open up insights contained in these datasets (while maintaining privacy) to support the design, implementation, monitoring and evaluation of development and humanitarian initiatives. To date, no such ‘techno-political’ system has been deployed at scale.
OPAL came out of the recognition that accessing Big Data sources for research and policy purposes presents a conundrum. To date, data held by private companies have been accessed externally either through data challenges, academic pilots, or through bilateral agreements. While these types of engagements have offered evidence of the data’s promise and clear user demand, they limit the full realization of Big Data’s social potential, as they fail to meet the standards that experimentations such as MIT’s OpenPDS and Bandicoot have shown to be both possible and essential: safe, stable, scalable access for public good purposes.
By ‘sending the code to the data’ rather than the other way around, OPAL seeks to address these challenges, spark dialogues and develop data services on the basis of greater trust.
OPAL came out of the recognition that accessing Big Data sources for research and policy purposes presents a conundrum. To date, data held by private companies have been accessed externally either through data challenges, academic pilots, or through bilateral agreements. While these types of engagements have offered evidence of the data’s promise and clear user demand, they limit the full realization of Big Data’s social potential, as they fail to meet the standards that experimentations such as MIT’s OpenPDS and Bandicoot have shown to be both possible and essential: safe, stable, scalable access for public good purposes.
By ‘sending the code to the data’ rather than the other way around, OPAL seeks to address these challenges, spark dialogues and develop data services on the basis of greater trust.
OPAL in Action
OPAL pilot initiatives are currently in development for National Statistical Offices (NSO) in Mexico and Senegal to gain access to non-traditional data sources. This community of stakeholders consists of influential, data-literate actors that can lay the foundations for expanding the project. As NSOs are in contact with other national ministries, they are well positioned to drive change in countries of the Global South. Typically limited to specific types of traditional data in their statistical analyses, NSOs are in critical need of new data and more efficient ways to measure indicators and build capacity.
Mission Statement
To help shape future technological, political, ethical, and legal frameworks and principles that will govern the local collection, control, and use of ‘sensitive data’ to foster social progress. Value creation happens for many parties in OPAL; this model includes data providers, algorithm providers, and a network of sustainable Data Services.
OPAL’s technical core consists of an open platform and set of certified algorithms that can be run on the data centers of partner organizations, behind their firewalls, to extract key indicators deemed relevant and safe by local stakeholders for a wide range of potential use cases, including in crisis contexts.
OPAL will be used to engage with data providers, users, and analysts at all stages of its development, to build local capacities and connections. In other words, it aims to help crack one of the Data Revolution’s main conundrums, which has been to extract value from personal data without infringing on individual and group privacy and agency –especially in contexts and times of heightened vulnerabilities and imbalances of power.
Real World Applications
OPAL and its technology have already proven effective in a number of ongoing projects. A brief summary of these applications is as follows:
Climate-Induced Migration in Africa and Beyond: Big Data and Predictive Analytics (CLIMB)
In Senegal, as part of a project funded by the Belmont Forum titled “Climate-Induced Migration in Africa and Beyond: Big Data and Predictive Analytics” (CLIMB), DPA and its academic and civil society partners will be utilizing ‘traditional’ and ‘non-traditional’ data, including mobile phone data, to understand human mobility induced by extreme weather events. In addition to Call Detail Records (CDR) provided through a partnership with Orange-Sonatel to study mobility patterns at a high resolution, the CLIMB project will leverage earth observation (EO) and social media data, and combine them with survey and official statistical data. This holistic approach will allow us to analyze migration processes from a multi-stage perspective (e.g. from internal displacement to onward/return migration), and gain more insights about the temporality of climate-induced migration. It will also allow us to better understand how migratory processes are shaped by multi-level (macro, meso, and micro) factors, including climate related, social, economic, sociological and cultural.
Big Data Projects for SDG Monitoring in Morocco
In Morocco, in collaboration with the United Nations Development Program (UNDP) and Orange, we are currently exploring the feasibility of using CDRs to predict and prevent breakouts of vector-borne diseases such as tuberculosis, malaria, or COVID-19 through an analysis of human mobility patterns and the spatial identification of the sources of contamination. In the case of malaria, our team in Morocco is seeking to replicate the methodology of a multidisciplinary study carried out by institutions in the United States and England (Ruktanonchai et al., 2016 ) for tuberculosis. This study showed how mobile phone mobility data could be used to conduct a network analysis of regions according to their human mobility and to identify sinks for breeding mosquitoes that transmit the disease, allowing policymakers to target the more vulnerable areas. According to the Ministry of Health that is participating actively in the project, this disease affects currently around 30,000 people.
Another OPAL-enabled initiative in Morocco is working to estimate the suicide mortality rate measured by the indicator 3.4.2 of the SDGs. Orange data will help identify mobility patterns of human behavior related to depression and use these results as an input of the indicator 3.4.2. The methodology used to estimate this indicator could be adapted to the context of various African countries.
Monitoring and Mapping Multidimensional Poverty in Brazilian Favelas
In collaboration with MIT and the Brazilian NGO Gerando Falcões (GF), we are exploring a partnership with the mobile network operator (MNO) TIM Brazil, to use CDRs to map and monitor poverty in 2 large favelas. Building on local knowledge from our partner GF, we will construct a Multidimensional Poverty Index that leverages such ‘non-traditional’ data to build a replicable, scalable, low-cost, real-time monitoring system. The project follows the methodology of Luo et al. (2022), adapting its sources and foci to the local context of the selected favelas, while also maintaining the holistic vision of the multiple deprivations of living in poverty. The methodology will also be scalable to other favelas and can be adapted to virtually any informal settlement globally.
Resources
Official statistics and big data in Latin America: Data enclosures and counter-movements
- Arruda d’Alva, O. and Paraná, Edemilson
- Journal Article
- 2024
With the rise of the data-driven economy, the state-owned sector of official statistics has been pressured to ‘modernise’ and engage with Big Data. However, most of these data sources are controlled by tech corporations. This journal article address the conflicts this brings about from a Latin American perspective through three case studies—including OPAL—, involving national statistical offices, international organizations, and the private sector.