LINKS WE LIKE #39
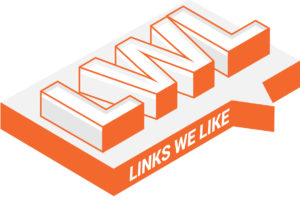
The Data Police: How AI and Big Data are Reshaping Crime Prevention
Imagine being able to detect crime hotspots in your city, use facial recognition software to match camera footage from a robbery with a database of images, or even predict the likelihood of a crime (including time and location) before it occurs. While this might sound like science fiction (Minority Report, which came out 20 years ago, of course comes to mind), these are just a few current examples of how Big Data and AI are currently being used to fight crime. One of the first uses of these technologies for crime prevention was within the Los Angeles Police Department in 2010, after they discovered that crime followed similar patterns, much like the aftershocks of an earthquake. Based on this observation, the LAPD developed a mathematical model using crime data to create an algorithm that was able to uncover patterns in the occurrence of crimes. Today, police departments around the world continue to do this, utilizing much more precise and accurate tools, (predpol, shotspotter, IBM i2 Coplink, Microsoft Azure Data Lake and Watson Analytics) that learn from large datasets, including police records, surveillance cameras and other sources to predict crime before it happens. These technologies have not only proven effective in predicting property crime, but also in preventing terrorist attacks, and tracking down sex offenders and financial criminals. Some results have been impressive, as in the UK, where digital crime maps are considered to be 10 times more effective at predicting crime than police officers. In a recently published book on the subject, there is a summary of the major ways in which Machine Learning is (and will be) used to understand, predict, and perhaps even prevent crime. However, critics of these approaches such as Catherine O’Neil, in her 2016 book “Weapons of Math Destruction”, have raised compelling concerns.
Replicating Discriminatory Patterns?
You may wonder, with such promise and potential for Big Data and AI to revolutionize criminal justice, what’s the catch? The answer lies in the various ethical implications that these technologies present, including the potential replication of discrimination. One of the aspects that causes the most concern is the risk that these predictive algorithmic systems will replicate racially-biased outcomes, as they are trained on historic crime data (which tends to be biased). This could, in turn, cause police officers to congregate in certain “high-crime” areas, leading to more stigmatization (and policing) of the populations living there. This issue gets even more complex when it comes to facial recognition. According to the National Association for the Advancement of Colored People (NAACP), in the USA, Black people are 5 times more likely to be stopped by the police than White people. Additionally, 56% of the incarcerated population are either Black or Latino. These statistics matter because it means that Black and Latino individuals are far more likely to have mugshots and other information stored in police databases, which are then used for cross-referencing with facial recognition data. One frightening consequence of this imbalance includes misidentification, which can lead to wrongful arrest. According to the New York Times, this exact scenario occurred in 2020 when three Black men were wrongfully arrested after being mistakenly identified by facial recognition software.
Privacy Concerns
In addition to bias replication, facial recognition software also has privacy implications. Companies like Clearview AI, which helps law enforcement agencies with photo-matching, have recently come under scrutiny for their lack of transparency and other security flaws. They operate by collecting millions of photos taken from social media and other internet sources, without users’ consent, and selling them to law enforcement agencies. Despite the common argument that someone who has “nothing to hide” shouldn’t mind sharing their data for the “greater good”, having personal data at the disposal of companies or police departments can have serious repercussions, including (but not limited to) wrongful identification, unlawful surveillance, and possible security breaches (which happened to Clearview AI in 2020) that can lead to identity theft. These privacy concerns become even more serious under authoritative regimes, where activists and academics have linked surveillance tools to spyware programs meant to track and target those who oppose the government. An investigation conducted by The Wall Street Journal discovered that Ugandan intelligence officials used spyware to intercept communications from opposition leader Bobi Wine, providing a real-world example of this kind of repression. Taken together, despite the promises of Big Data and AI to fight crime, the use of these technologies must be carefully evaluated and regulated to protect citizens’ physical security and privacy. To achieve this, a series of measures, such as the regulation of surveillance and AI technologies, ethical supervision boards, continuous algorithmic revision, and privacy protection laws must be put into place to ensure that these tools are applied as fairly and ethically as possible.
Join us in the edition of Links We Like as we explore the applications and implications of using Big Data and AI to predict, measure, and fight crime.
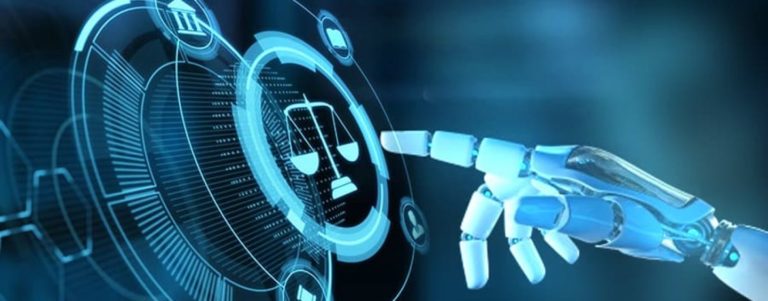
El big data es cada vez más utilizado para mejorar servicios públicos, entre ellos la prevención del crimen. Ante la actual presencia de miles de datos digitales, la Policía Digital h50 explora dos herramientas que permiten potencializar el big data para combatir el crimen. Una de ellas es PredPol (The Predictive Policing Company) que con base en las denuncias a la policía y los índices de victimización, permite predecir dónde y cuándo es más probable que ocurran los crímenes. Esto permite una mejor asignación de fuerzas policiales y de recursos, cerrando la brecha de información con datos y reportes. Beware es otro sistema que se utiliza en California para predecir la delincuencia con base en datos de redes sociales. Finalmente, h50 resalta la creación por parte de IBM del sistema Watson, que ha aprendido el “lenguaje de la ciberseguridad” y es capaz de detectar riesgos y valorar posibles amenazas de ataques cibernéticos. Varios de estos sistemas han sido cuestionados por los desafíos éticos que representan, como el riesgo de profile o “encasillar” a ciertos grupos de personas con base en estereotipos. Sin embargo, de lograr superar estos riesgos, estas tecnologías permiten hacer un mayor y mejor uso del big data para potenciar la seguridad.
O uso de Big Data e Inteligência Artificial tem se mostrado promissor no combate e prevenção ao crime nos últimos anos. No Brasil, apesar de muitas iniciativas ainda se encontrarem em fase de desenvolvimento, soluções inovadoras contra o crime construídas a partir do cruzamento de dados já têm sido implementadas com sucesso. Por exemplo, a Universidade Federal do Ceará e a Secretaria de Segurança Pública do estado desenvolveram 9 projetos de combate ao crime a partir de 60 fontes de dados ligados à segurança pública. Estes projetos funcionam a partir do uso da linguagem natural, sistema automatizado de busca de impressões digitais e detector de marcas e modelos de veículos a partir de uma ampla base de imagens (entre outras ferramentas), permitindo que policiais e secretarias de combate ao crime consigam identificar criminosos com mais facilidade e rapidez. Soluções semelhantes também podem ser vistas no combate à fraude, um crime que tem crescido exponencialmente com a rápida evolução dos meios de comunicação pela internet. A fim de prevenir cibercrimes, diversas empresas e instituições apostam na utilização de ferramentas de Big Data e Machine Learning para prever possíveis falhas de segurança e calcular o risco de fraude. Um exemplo deste tipo de ferramenta é a plataforma Konduto, que analisa o comportamento de navegação e compra do individuo para calcular o risco de fraude em uma possível transação bancária virtual.
Technology based on Artificial Intelligence is now being used to identify criminal suspects. In this Wired podcast, the hosts discuss what happens when mistakes are made and things go horribly wrong (for example, the arrest and conviction of an innocent person). Unsurprisingly, those that end up facing the most negative consequences from computer misidentification often belong to vulnerable populations, such as women, Black men and youth. The limitations of facial recognition have repercussions on people’s lives everyday, so go ahead and listen to this episode of “Gadget Lab” for a comprehensive, nuanced, and interesting discussion of the timely topic.
Data and social scientists from the University of Chicago, utilizing publicly available data on violent and property crimes, have developed an algorithm to forecast future crimes. By learning from the time and geographical locations revealed by this data, the algorithm can “predict” future crimes one week before they happen, with up to 90% accuracy. The model is able to achieve such remarkable results by isolating crime by looking at the time and spatial coordinates of crime events to detect patterns. Subsequently, the city is divided into 1,000 ft. wide spatial “tiles”, rather than relying on traditional political boundaries, which can be subject to bias. However, one of the algorithm’s creators, Ishanu Chatoopadhyay, PHD cautioned that even though the algorithm achieved such accurate predictive results, it should not be used by law enforcement to “swarm” an area where a crime may occur, but rather as part of the “tool kit” of urban policing.
Further Afield
Big data and AI for crime prevention
- Computational Approaches to the Study of Corruption
- Combating crime with Big data, analytics and artificial intelligence
- Surveillance and Predictive Policing Through AI
- How big data analytics helps in crime prediction and prevention
- Big Data e Inteligência Artificial em ação no combate ao crime
- Big Data e Policiamento Preditivo | BigDataCorp.
- What Happens When Police Use AI to Predict and Prevent Crime?
- Using Artificial Intelligence to Address Criminal Justice Needs
- The Never-ending Quest to Predict Crime Using AI
- 5 Ways Big Data Can Change Crime Prevention
- Predictive Policing: Big Potential as Big Data Fights Crime
- ¿Se puede combatir el crimen con el Big Data?
- Luchar contra el crimen con big data
- Big data y prevención del crimen: en la ficción y en la realidad
- Automated Justice: Algorithms, Big Data and Criminal Justice Systems
Examples of Big data and AI as tools to fight crime
- Using Big Data Analytics to Combat White-Collar Crime
- This Secret $35 Million FBI Unit Mixes Facial Recognition With Big Data To Investigate America’s Most Horrific Crimes
- Socio-economic, built environment, and mobility conditions associated with crime: A study of multiple cities
- AI predicts crime a week in advance with 90 per cent accuracy
- Aplicativo vai agilizar atendimento policial para vítimas de violência doméstica | Ceará | G1
- Big Data permite indicar crimes cruzando dados existentes
- Big data no combate à fraude – Tail Blog
- Como usar Big Data para barrar fraudes na internet?
- After Uvalde: Could AI Prevent Another School Shooting?
- Japanese Municipalities Turn to AI for Crime Prevention
- The Case for Placing AI at the Center of Digitally Robust Financial Regulation
- La utilidad del big data para la prevención del crimen
- El big data como arma para combatir el crimen
- El big data como herramienta para la prevención de la delincuencia
- Security tool guarantees privacy in surveillance footage
Risks of using Big Data and AI for crime prevention
- Weapons of Math Destruction
- The danger of predictive algorithms in criminal justice | Hany Farid | TEDxAmoskeagMillyard
- ‘An Invisible Cage’: How China Is Policing the Future
- What Data About You Can the Government Get From Big Tech?
- The Slippery Slope of Big Data in Policing
- A data ‘black hole’: Europol ordered to delete vast store of personal data
- Africa: regulate surveillance technologies and personal data
- The AI Placed You at the Crime Scene, but You Weren’t There
- Artificial intelligence in criminal justice: invasion or revolution?
- Researchers use AI to predict crime, biased policing in major U.S. cities like L.A.