LINKS WE LIKE #24
We are currently living in the age of information and data. Whether it be through news outlets or social media, everyday we are bombarded with hundreds of images and headlines that come in the shape of articles, personal stories, memes or videos. In this busy landscape, “fake news” and misinformation have become an increasing tendency, almost the “new normal”, with far-reaching consequences including impacting the results of the 2016 United States election and, more recently, the spreading of false information around the COVID-19 pandemic.
To counter these issues, many fact-checking initiatives (e.g. Fact Check, Politifact, The Trust Project and Snopes) have emerged to help identify when a post, article or video contains dubious or fake content. However, this task – which usually requires careful human inspection -is a slow and inefficient process, especially in terms of preventing false information from going viral. In that regard, Facebook’s strategy is interesting, if lacking. The company recently added a tool that allows users to flag news-feed posts as “fake news”. When a large number of people do this, the post appears on other users’ feed with less frequency and carries a warning. Unfortunately, user-generated reporting can backfire and lead to censorship, as experts believe has been the case for the Syrian government opposition. In this context of socio-economic polarization, lack of regulation and low will from the private sector, “fake news” (which are also easy to create) continue to proliferate. Moreover, since this kind of content is not only produced by humans, but also by bots and Artificial Intelligence, identifying, controlling and tracking misinformation has become a complex and surmounting endeavour.
Big Data and Artificial Intelligence approaches have been leveraged to tackle this phenomenon by creating models to automatically decipher false information and prevent it from spreading. For example, professor Maite Taboada from Simon Fraser University has focused her research in making social media and online-platform discussions a more reliable and safe space for communication by working at the intersection of linguistics, computational linguistics and data science fields. However, Machine Learning projects have not all been used to fight misinformation, but rather in some cases – as what happened with the telecom provider Vittel–, to facilitate the propagation of deep-fake videos or the use of OpenAI GPT-2 to automatize “fake news”.
To explore the danger of misinformation and the tools and strategies being used to stop them from propagating, this edition of Links We Like addresses the topic by exploring some of the key challenges, limitations and opportunities brought forward by this developing field of work.
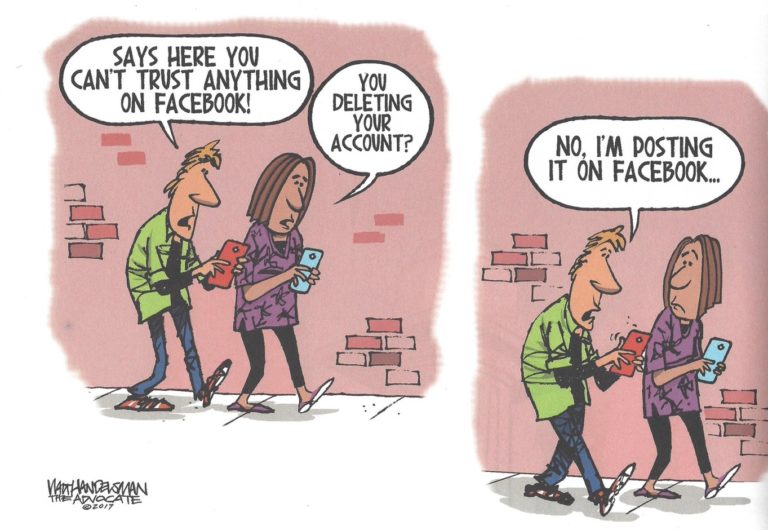
Louisiana State University
In this episode of the Data Journalism podcast, Buzzfeed’s media editor Craig Silverman talks about online misinformation and the importance of content verification in journalists’ work. In this conversation, Craig argues that verification processes are necessary to assess that the news and data we receive has been created by ‘reliable’ sources. He also points to the second Verification Handbook published in 2020 where leading practitioners in the area of disinformation and media manipulation comment on the current information challenges faced worldwide. At last, he makes a call for newsrooms to question their sense of urgency in publishing news and to raise the bar by cultivating verification skills.
In an interview with The Economist, misinformation specialist Samuel Wooley discusses his latest book regarding the role of technology in creating and spreading misinformation and hate speech. In his book, he argues that AI – such as ‘deep fake videos’ bots and machine learning programs are permeating the digital sphere, creating confusion among users and endangering democratic practices. However, the solution to the problem also requires AI technologies to automatically identify false information or hate speech in articulation with a stronger educational platform for media literacy. Furthermore, to confront the larger issue, big companies like Facebook and Twitter need to implement more rigorous policies regarding information operations and hate speech.
By conducting one of the largest studies on fake news (which encompassed analyzing around 126,000 stories tweeted by 3 million users for over more than 10 years), MIT professors Sinan Aral, Deb Roy and Darthmouth professor Soroush Vosoughi observed that false news were far more likely to reach more people than the truth. In their study, they found that a false story on average reaches 1,500 people six times quicker than a true story, and that the top 1% of false news is diffused to around 1,000 and 100,000 people while the truth is rarely diffused to more than 1,000 people. Additionally, the authors found out that tweets spread by bots and by real users were retweeted at the same rate, and furthermore through a sentiment-analysis tool, they found that the spread of false content on Twitter is greatly influenced by the appeal in the language used by ‘fake news’, which usually evokes feelings of surprise and disgust.
Funded by the European Union, the We Verify project tackles advanced content verification through a participatory verification approach, open source algorithms and machine learning. The project will analyse social media and web content to detect misinformation and then proceed to expose any misleading or fabricated content by micro-targeted debunking and a blockchain-based public database of publications that are known to be fake. Due to its open source nature, the platform engages communities, newsrooms and journalists to enable in-house content management systems while having the support of digital tools to assist with verification tasks. Furthermore, the platform developed a browser plugin to facilitate advanced content verification tools to easily fact-check information.
A team from the MIT Computer Science & Artificial Intelligence Lab developed a research project where they questioned previous fake news identifiers that relied on automatic text detectors of machine-generated text created with OpenAI’s GPT-2 language model. Parting from the idea that the most intrinsic characteristic of fake news is their factual falseness and not if the information was human or machine generated, the team calls into question the credibility of current misinformation classifiers. Based on these findings, the team developed a system to detect false statements using the world’s largest fact-checking dataset, Fact Extraction and VERification (FEVER). Although at first the created model focused too much on the language of the text and not the external factual evidence, they later created an algorithm that outperformed previous ones in all metrics.
Further Afield
Screenshot from National Geographic page
Fake news and the verification of information
- Knowledge-Based Trust: Estimating the Trustworthiness of Web Sources
- Digital verification for human rights advocacy
- Big Data and quality data for fake news and misinformation detection
AI tackling misinformation
- Artificial Intelligence and Disinformation
- Using AI to combat fake news
- Identifying, Tracking, and Fact-Checking Misinformation
- Facebook using AI to help detect misinformation
AI and the spread of misinformation